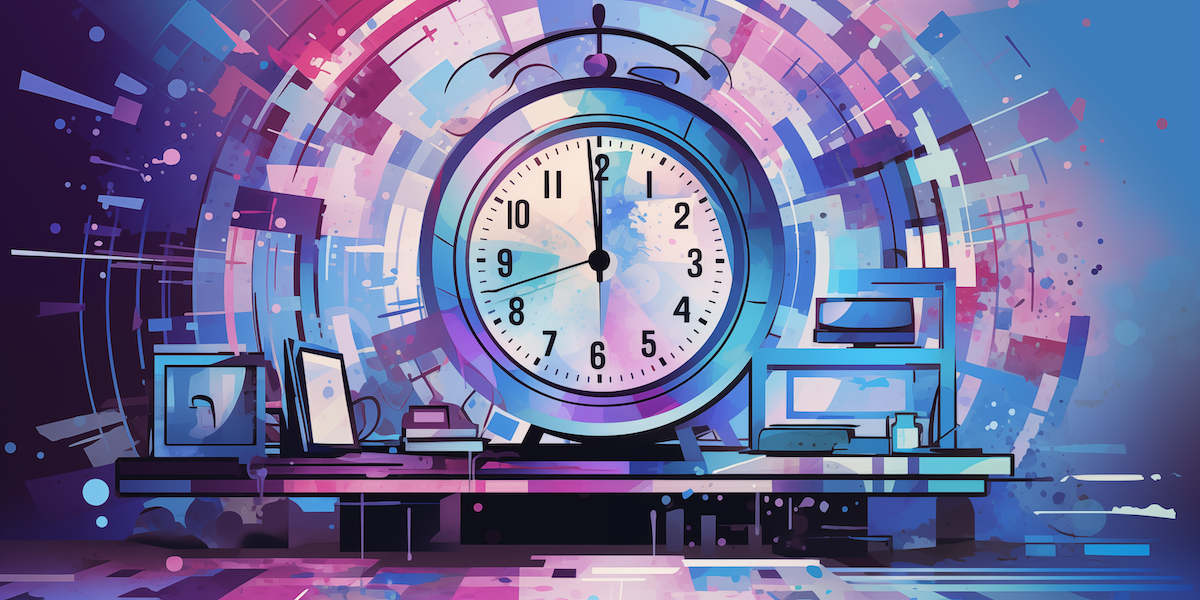
Risk adjustment leaders face mounting challenges to deliver more with less. At every industry conference, the promises for AI to help seem to grow bigger and bolder. Yet beneath the hype, there's at least one real shift happening: the latest generation of AI for risk adjustment is delivering measurable results, starting with retrospective reviews.
Last month at RISE West in Colorado Springs, I joined industry veteran RaeAnn Grossman–formerly of Molina, Cotiviti, Datavant, and Signify–to discuss exactly this reality. From this discussion and my ongoing conversations with health care leaders, one thing is clear: the latest generation of AI in risk-adjustment coding is quickly becoming a competitive necessity.
Needless to say, today's market pressures are unprecedented: an aging population, expanding value-based care programs, and stricter OIG audit requirements. The v24 to v28 model transition has expanded HCC counts while reducing mappable ICDs, requiring weeks or months of retraining for manual coding teams. As a result, organizations must find ways to review charts more efficiently than ever while still meeting accuracy targets of 95 percent or higher.
So far, vendors aren't helping very much with these pressures. Consolidation has reduced traditional sources of capacity and put strain on experienced coders, while inflation has seen labor costs skyrocket. Health plan leaders are looking for solutions that can scale operations sustainably and increase speed without compromising quality. Questions from many leaders boil down to: How can we maintain accuracy and compliance while controlling costs and developing our workforce for the AI future?
To answer this question, let's zoom out and trace the recent history of technology for risk adjustment. The industry's first attempts at meaningful automation came through natural language processing (NLP) tools in the early 2000s. While these systems showed promise for simple cases, they struggled with even moderate complexity. The 2015 transition to ICD-10 exposed their limitations: When diagnosis codes ballooned from sets of 13,000 to 69,000, these rules-based systems simply couldn't adapt.
What is less familiar to many leaders is that a game-changer came in 2018, with the advent of deep learning AI. Unlike rigid, rules-based predecessors, this technology learns from hundreds of millions of coded examples to handle high complexity. Since early applications in 2021, deep-learning-based platforms have consistently exceeded human accuracy benchmarks in risk-adjustment coding.
To quantify the practical impact of this next generation of RA tech, consider the case of an early adopter. This health plan with two million members, including 400,000+ Medicare Advantage lives, implemented deep learning AI for retrospective reviews with striking results: 91.2 percent full chart automation, 38 percent fewer coding errors, and 27 percent increased ICD capture. Most significantly, this plan reduced vendor coding spend by 47.8 percent.
While some health plan leaders remain skeptical of AI for RA, citing concerns about oversight and the human element in coding, these objections miss today's reality. The latest generation of technology already exceeds average human accuracy, as early adopters have proven. And to win over compliance teams–who are best brought in at the very beginning of an evaluation process–strong vendor partners can demonstrate performance at scale by running side-by-side coding accuracy comparisons between technology and manual teams in a blinded fashion.
In a broader sense, this technological shift invites an exciting, once-in-a-generation career and organizational opportunity for health plan leaders. With the increasing pace of AI, the role of an executive is evolving from managing teams to managing software. In the case of RA coding, this is already happening as leaders implement new technology to handle the vast majority of the workload effectively, enabling skilled coders to focus on higher-value activities at the top of their license.
For health plan executives, the question isn't about whether to adopt the latest AI for risk-adjustment coding. It's about taking the oncoming pressures seriously and bolstering your organization with all available tools to handle them–now including deep learning.
About the author
Andrew Lockhart is CEO of Fathom, the leader in autonomous coding. Fathom applies cutting-edge deep learning to produce complete risk-adjustment coding results for health plans, managed care organizations, and health systems. Lockhart is a recognized industry expert, having published dozens of articles in leading industry publications such as Healthcare Business Today and HIT Consultant, while earning awards such as Slice of Healthcare's Top Digital Health Leader in 2023. He has presented to health care executives at Becker's, Google, Healthcare Finance Institute, Health Innovation Roundtable, and RISE, among other engagements. He earned his MBA from Stanford University and his BA from the University of Toronto.